Análisis de características tiempo-frecuencia para la predicción de series temporales de Material Particulado usando Regresión por Vectores de Soporte y Optimización por Enjambre de Partículas
Time-Frequency characteristics analysis for forecasting time series of particulate matter using Support Vector Regression and Particle Swarm Optimization
Barra lateral del artículo
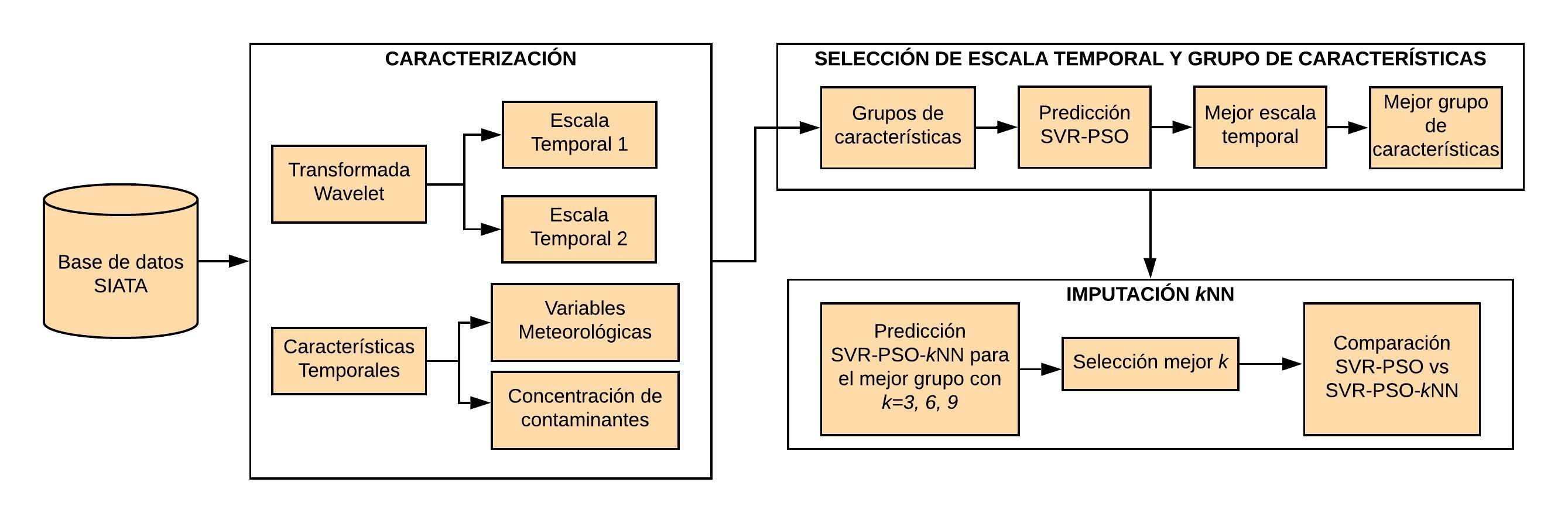
Términos de la licencia (VER)

Esta obra está bajo una licencia internacional Creative Commons Atribución-NoComercial-SinDerivadas 4.0.
Declaración del copyright
Los autores ceden en exclusiva a la Universidad EIA, con facultad de cesión a terceros, todos los derechos de explotación que deriven de los trabajos que sean aceptados para su publicación en la Revista EIA, así como en cualquier producto derivados de la misma y, en particular, los de reproducción, distribución, comunicación pública (incluida la puesta a disposición interactiva) y transformación (incluidas la adaptación, la modificación y, en su caso, la traducción), para todas las modalidades de explotación (a título enunciativo y no limitativo: en formato papel, electrónico, on-line, soporte informático o audiovisual, así como en cualquier otro formato, incluso con finalidad promocional o publicitaria y/o para la realización de productos derivados), para un ámbito territorial mundial y para toda la duración legal de los derechos prevista en el vigente texto difundido de la Ley de Propiedad Intelectual. Esta cesión la realizarán los autores sin derecho a ningún tipo de remuneración o indemnización.
La autorización conferida a la Revista EIA estará vigente a partir de la fecha en que se incluye en el volumen y número respectivo en el Sistema Open Journal Systems de la Revista EIA, así como en las diferentes bases e índices de datos en que se encuentra indexada la publicación.
Todos los contenidos de la Revista EIA, están publicados bajo la Licencia Creative Commons Atribución-NoComercial-NoDerivativa 4.0 Internacional
Licencia
Esta obra está bajo una Licencia Creative Commons Atribución-NoComercial-NoDerivativa 4.0 Internacional
Contenido principal del artículo
Resumen
La contaminación atmosférica por Material Particulado (PM) es un problema claramente reconocido a nivel mundial como uno de los factores de riesgo más importantes para la salud humana, en los últimos años han surgido diferentes modelos basados en inteligencia artificial para predecir la concentración de PM, con el fin de generar sistemas de alerta temprana que eviten la exposición de las personas. En este trabajo, se analizó un esquema de caracterización en el dominio tiempo-frecuencia usando la transformada Wavelet para la predicción de series temporales de PM10 y PM2.5 usando un algoritmo de Regresión por Vectores de Soporte optimizado por Enjambre de Partículas (SVR-PSO), además, se evaluó el efecto de la imputación de datos sobre las estimaciones. Los resultados obtenidos mostraron que, empleando características temporales, más las características tiempo-frecuencia propuestas, se obtiene el mejor desempeño de la SVR-PSO, además se encontró que el uso de la imputación de datos no afecta el desempeño de la SVR-PSO. El sistema propuesto en este trabajo permite disminuir el error de las estimaciones de concentración de PM10 y PM2.5 haciendo uso de características tiempo-frecuencia y es capaz de operar de forma robusta contra datos perdidos, aumentando su viabilidad de ser implementado en escenarios reales.
Descargas
Detalles del artículo
Juan Pablo Sepulveda Suescun, Instituto Tecnológico Metropolitano
Ingenierio Biomédico.
Docente cátedra ITM de la facultad de ciencias exactas y aplicadas
Referencias (VER)
Ahmat Zainuri, N., Aziz Jemain, A. and Muda, N. (2015) ‘A Comparison of Various Imputation Methods for Missing Values in Air Quality Data (Perbandingan Pelbagai Kaedah Imputasi bagi Data Lenyap untuk Data Kualiti Udara)’, Sains Malaysiana, 44(3), pp. 449–456. Available at: http://www.ukm.edu.my/jsm/pdf_files/SM-PDF-44-3-2015/17 NuryAzmin.pdf.
Araghi, A. et al. (2015) ‘Using wavelet transforms to estimate surface temperature trends and dominant periodicities in Iran based on gridded reanalysis data’, Atmospheric Research. Elsevier B.V., 155, pp. 52–72. doi: 10.1016/j.atmosres.2014.11.016.
Bai, L. et al. (2018) ‘Air pollution forecasts: An overview’, International Journal of Environmental Research and Public Health, 15(4), pp. 1–44. doi: 10.3390/ijerph15040780.
Baklanov, A. et al. (2007) ‘Integrated systems for forecasting urban meteorology, air pollution and population exposure’, Atmospheric Chemistry and Physics, 7(3), pp. 855–874. doi: 10.5194/acp-7-855-2007.
Betancur Alarcon, L. (2017) ‘Atencion de males por calidad del aire cuesta 1,6 billones al año’, El Tiempo, May.
Brugha, R., Edmondson, C. and Davies, J. C. (2018) ‘Outdoor air pollution and cystic fibrosis’, Paediatric Respiratory Reviews, 28, pp. 80–86. doi: https://doi.org/10.1016/j.prrv.2018.03.005.
Chen, M. et al. (2015) ‘A clustering algorithm for sample data based on environmental pollution characteristics’, Atmospheric Environment. Elsevier Ltd, 107, pp. 194–203. doi: 10.1016/j.atmosenv.2015.02.042.
Chen, Y. et al. (2013) ‘Ensemble and enhanced PM10 concentration forecast model based on stepwise regression and wavelet analysis’, Atmospheric Environment. Elsevier Ltd, 74, pp. 346–359. doi: 10.1016/j.atmosenv.2013.04.002.
Delpont, B. et al. (2018) ‘Environmental Air Pollution: An Emerging Risk Factor for Stroke’, in Vasan, R. S. and Sawyer, D. B. (eds) Encyclopedia of Cardiovascular Research and Medicine. Oxford: Elsevier, pp. 231–237. doi: https://doi.org/10.1016/B978-0-12-809657-4.99588-7.
Donnelly, A., Misstear, B. and Broderick, B. (2015) ‘Real time air quality forecasting using integrated parametric and non-parametric regression techniques’, Atmospheric Environment. Elsevier Ltd, 103(2), pp. 53–65. doi: 10.1016/j.atmosenv.2014.12.011.
Ertuugrul, Ö. F. and Taugluk, M. E. (2017) ‘A novel version of k nearest neighbor: Dependent nearest neighbor’, Applied Soft Computing Journal, 55, pp. 480–490. doi: 10.1016/j.asoc.2017.02.020.
Feng, X. et al. (2015) ‘Artificial neural networks forecasting of PM2.5 pollution using air mass trajectory based geographic model and wavelet transformation’, Atmospheric Environment, 107, pp. 118–128. doi: 10.1016/j.atmosenv.2015.02.030.
Gallego, A. J. et al. (2018) ‘Clustering-based k-nearest neighbor classification for large-scale data with neural codes representation’, Pattern Recognition. Elsevier Ltd, 74, pp. 531–543. doi: 10.1016/j.patcog.2017.09.038.
García Nieto, P. J. et al. (2017) ‘Air Quality Modeling Using the PSO-SVM-Based Approach, MLP Neural Network, and M5 Model Tree in the Metropolitan Area of Oviedo (Northern Spain)’, Environmental Modeling & Assessment. doi: 10.1007/s10666-017-9578-y.
De Gennaro, G. et al. (2013) ‘Neural network model for the prediction of PM10 daily concentrations in two sites in the Western Mediterranean’, Science of the Total Environment. Elsevier B.V., 463–464, pp. 875–883. doi: 10.1016/j.scitotenv.2013.06.093.
Hu, C. et al. (2014) ‘Data-driven method based on particle swarm optimization and k-nearest neighbor regression for estimating capacity of lithium-ion battery’, Applied Energy. Elsevier Ltd, 129, pp. 49–55. doi: 10.1016/j.apenergy.2014.04.077.
Hu, X. P., Dong, X. D. and Yu, B. H. (2016) ‘Method of Optimal Design with SVR-PSO for Ultrasonic Cutter Assembly’, Procedia CIRP, 50, pp. 779–783. doi: 10.1016/j.procir.2016.04.180.
Kalteh, A. M. (2015) ‘Wavelet Genetic Algorithm-Support Vector Regression (Wavelet GA-SVR) for Monthly Flow Forecasting’, Water Resources Management, 29(4), pp. 1283–1293. doi: 10.1007/s11269-014-0873-y.
Kazem, A. et al. (2013) ‘Support vector regression with chaos-based firefly algorithm for stock market price forecasting’, in Applied Soft Computing. Elsevier B.V., pp. 947–958. doi: 10.1016/j.asoc.2012.09.024.
Khaniabadi, Y. O. et al. (2018) ‘Mortality and morbidity due to ambient air pollution in Iran’, Clinical Epidemiology and Global Health. doi: https://doi.org/10.1016/j.cegh.2018.06.006.
LINDSAY, P. H. and NORMAN, D. A. (1977) ‘Neural information processing’, Human Information Processing, 8226(November), pp. 190–254. doi: 10.1016/B978-0-12-450960-3.50010-5.
Marini, F. and Walczak, B. (2015) ‘Particle swarm optimization (PSO). A tutorial’, Chemometrics and Intelligent Laboratory Systems. Elsevier B.V., 149, pp. 153–165. doi: 10.1016/j.chemolab.2015.08.020.
Martínez, J. and Castro, R. (2002) ‘Análisis de la teoría ondículas orientada a las aplicaciones en ingeniería eléctrica:Fundamentos’, E.T.D.I. Industriales Dpt. de ingeniería eléctrica, p. 161.
Muñoz, A., Quiroz, C. and Paz, J. (2006) Efectos de la contaminación atmosférica sobre la salud en adultos. Universidad de Antioquia.
Murillo-Escobar, J. et al. (2019) ‘Forecasting concentrations of air pollutants using support vector regression improved with particle swarm optimization: Case study in Aburrá Valley, Colombia’, Urban Climate. Elsevier, 29(March), p. 100473. doi: 10.1016/j.uclim.2019.100473.
Partal, T. and Küçük, M. (2006) ‘Long-term trend analysis using discrete wavelet components of annual precipitations measurements in Marmara region (Turkey)’, Physics and Chemistry of the Earth, 31(18), pp. 1189–1200. doi: 10.1016/j.pce.2006.04.043.
Prasad, K., Gorai, A. K. and Goyal, P. (2016) ‘Development of ANFIS models for air quality forecasting and input optimization for reducing the computational cost and time’, Atmospheric Environment. Elsevier Ltd, 128, pp. 246–262. doi: 10.1016/j.atmosenv.2016.01.007.
Qin, S. et al. (2014) ‘Analysis and forecasting of the particulate matter (PM) concentration levels over four major cities of China using hybrid models’, Atmospheric Environment. Elsevier Ltd, 98, pp. 665–675. doi: 10.1016/j.atmosenv.2014.09.046.
Schraufnagel, D. E. et al. (2018) ‘Air Pollution and Noncommunicable Diseases: A Review by the Forum of International Respiratory Societies’ Environmental Committee, Part 2: Air Pollution and Organ Systems’, Chest. doi: https://doi.org/10.1016/j.chest.2018.10.041.
Shahraiyni, H. T. and Sodoudi, S. (2016) ‘Statistical modeling approaches for pm10 prediction in urban areas; A review of 21st-century studies’, Atmosphere, 7(2), pp. 10–13. doi: 10.3390/atmos7020015.
Shen, C. H., Huang, Y. and Yan, Y. N. (2016) ‘An analysis of multifractal characteristics of API time series in Nanjing, China’, Physica A: Statistical Mechanics and its Applications. Elsevier B.V., 451(June 2000), pp. 171–179. doi: 10.1016/j.physa.2016.01.061.
Siata (2017) Estabilidad atmosférica en el Valle de Áburra. Colombia.
Smola, a J. and Scholkopf, B. (2004) ‘A tutorial on support vector regression’, Statistics and Computing, 14(3), pp. 199–222. doi: Doi 10.1023/B:Stco.0000035301.49549.88.
Sun, W. et al. (2013) ‘Prediction of 24-hour-average PM2.5 concentrations using a hidden Markov model with different emission distributions in Northern California’, Science of the Total Environment. Elsevier B.V., 443, pp. 93–103. doi: 10.1016/j.scitotenv.2012.10.070.
Zhang, Y. et al. (2012) ‘Real-time air quality forecasting, Part II: State of the science, current research needs, and future prospects’, Atmospheric Environment. Elsevier Ltd, 60, pp. 656–676. doi: 10.1016/j.atmosenv.2012.02.041.
Zhang, Z. et al. (2017) ‘Application of a novel hybrid method for spatiotemporal data imputation: A case study of the Minqin County groundwater level’, Journal of Hydrology. Elsevier B.V., 553, pp. 384–397. doi: 10.1016/j.jhydrol.2017.07.053.
Artículos similares
- Jairo Armando Cardona Bedoya, Huberney Celemín Sanchez, Alvaro Pulzara Mora, Estudio de la influencia de la segregación de indio y del campo eléctrico interno en las propiedades ópticas de heteroestructuras de pozos cuánticos III-V , Revista EIA: Vol. 18 Núm. 36 (2021):
También puede {advancedSearchLink} para este artículo.